The Most Influential Machine Learning Trends Right Now
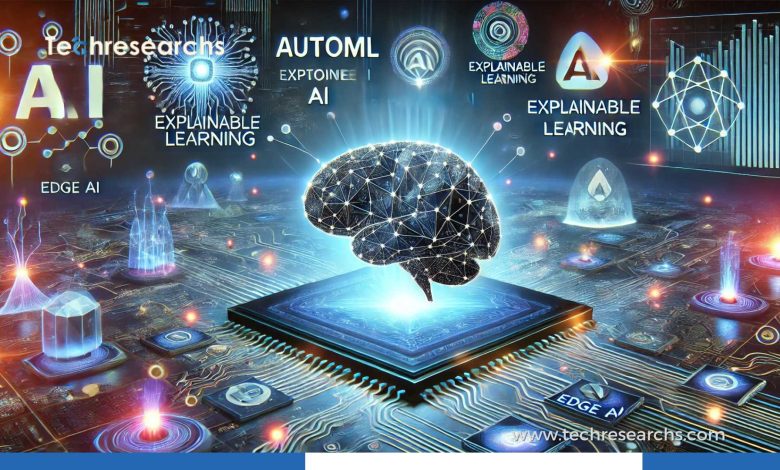
Machine learning is revolutionizing industries worldwide, driving automation, enhancing decision-making, and transforming business operations. As technology advances, machine learning trends continue to evolve, shaping the future of artificial intelligence (AI). In this article, we explore the most influential machine learning trends that are making a significant impact today.
1. Automated Machine Learning (AutoML)
Automated Machine Learning (AutoML) simplifies the process of developing machine learning models. It enables businesses to leverage AI without requiring extensive expertise. AutoML tools help automate data preprocessing, model selection, and hyperparameter tuning, making AI more accessible.
Key Benefits of AutoML:
- Reduces the need for expert data scientists
- Enhances model accuracy and efficiency
- Saves time in the AI development process
2. Explainable AI (XAI) for Transparency
As machine learning models become more complex, understanding their decision-making processes is crucial. Explainable AI (XAI) enhances transparency by providing insights into how algorithms make decisions. This trend is vital in sectors like healthcare and finance, where trust and compliance are essential.
Why XAI Matters:
- Increases trust in AI-driven decisions
- Enhances regulatory compliance
- Improves debugging and model refinement
3. Federated Learning for Data Privacy
Federated learning is a decentralized approach that enables AI models to train on data without transferring it to a central server. This method enhances privacy while maintaining model performance.
Applications of Federated Learning:
Industry | Use Case |
---|---|
Healthcare | Personalized medicine recommendations |
Finance | Fraud detection and risk assessment |
Retail | Personalized customer experiences |
4. AI-Powered Cybersecurity
With cyber threats evolving, machine learning is becoming essential for cybersecurity. AI-driven security systems detect anomalies, prevent cyberattacks, and respond to threats in real time.
How AI Enhances Cybersecurity:
- Detects unusual patterns and threats
- Improves response time to security incidents
- Automates risk management processes
5. Natural Language Processing (NLP) Advancements
Natural Language Processing (NLP) is improving the way machines understand and interact with human language. New AI models like GPT-4 and BERT are making NLP applications more effective.
Popular NLP Applications:
- Chatbots and virtual assistants
- Sentiment analysis for businesses
- Real-time language translation
6. Edge AI for Faster Processing
Edge AI moves AI computations closer to the data source, reducing latency and improving efficiency. This trend is crucial for real-time applications such as IoT devices, autonomous vehicles, and smart cameras.
Advantages of Edge AI:
- Reduces reliance on cloud computing
- Enhances real-time decision-making
- Lowers data transmission costs
7. Self-Supervised Learning
Traditional machine learning requires labelled data, which can be costly and time-consuming. Self-supervised learning enables models to learn from unlabelled data, making AI training more efficient.
Impact of Self-Supervised Learning:
- Reduces dependency on labelled datasets
- Speeds up AI model training
- Enhances AI’s adaptability to new tasks
8. AI in Drug Discovery and Healthcare
Machine learning is accelerating drug discovery and improving healthcare outcomes. AI-driven models analyse vast datasets to identify potential treatments and predict disease progression.
Examples of AI in Healthcare:
- Predicting patient outcomes
- Identifying new drug candidates
- Enhancing medical imaging analysis
9. Generative AI and Synthetic Data
Generative AI creates synthetic data that mimics real-world data, reducing privacy concerns and improving AI model training. This trend is beneficial in sectors like finance, healthcare, and gaming.
Use Cases of Generative AI:
- Creating realistic simulations
- Enhancing AI training with diverse datasets
- Reducing biases in machine learning models
10. Ethical AI and Bias Reduction
As AI adoption grows, addressing ethical concerns and reducing bias in AI models is crucial. Companies are focusing on developing responsible AI systems that prioritize fairness and accountability.
Steps Toward Ethical AI:
- Implementing fairness-aware algorithms
- Enhancing diversity in AI training data
- Ensuring transparency in AI decision-making
The rapid advancement of machine learning is transforming industries and driving innovation. From AutoML to Edge AI, these machine learning trends are shaping the future of technology. Staying informed about these trends can help businesses and individuals leverage AI effectively. As we move forward, ethical considerations and transparency will play a crucial role in AI development.
FAQs
Q1: What are the top machine learning trends today?
A: Some of the top machine learning trends include AutoML, Explainable AI, Federated Learning, AI in Cybersecurity, and Edge AI.
Q2: How does machine learning impact businesses?
A: Machine learning enhances decision-making, automates tasks, improves cybersecurity, and enables predictive analytics across various industries.
Q3: What is the importance of ethical AI?
A: Ethical AI ensures fairness, transparency, and accountability in machine learning models, reducing biases and improving public trust.
Q4: How is AI used in healthcare?
A: AI is used for disease diagnosis, drug discovery, personalized treatments, and predictive analytics to improve patient care.
Q5: What is self-supervised learning?
A: Self-supervised learning is an approach where AI learns from unlabelled data, reducing the need for manual data labelling.