What Are the Latest Solutions for Reducing AI Hallucination?
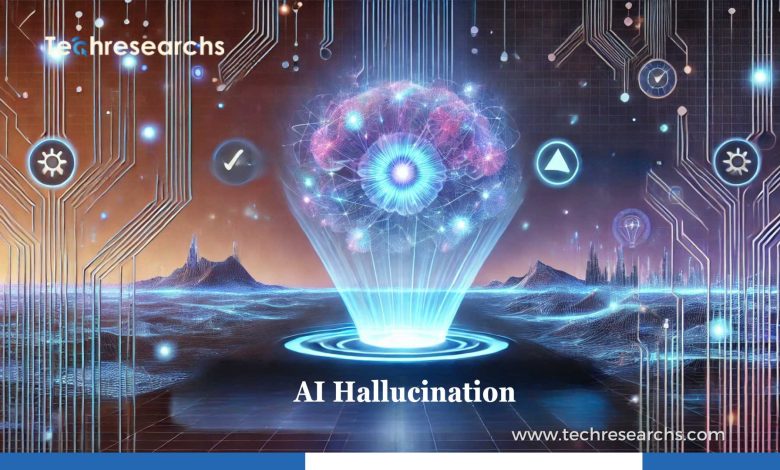
Artificial Intelligence (AI) has revolutionized various industries. However, one of its major challenges is AI Hallucination —when AI models generate false, misleading, or nonsensical information. This issue is particularly common in Large Language Models (LLMs) like GPT-4, which sometimes fabricate facts, citations, or responses.
As AI adoption grows, reducing AI hallucination is crucial for improving AI accuracy, reliability, and user trust. In this article, we explore the latest solutions to AI hallucination, their effectiveness, and how they are shaping the future of AI.
What Is AI Hallucination?
AI hallucination occurs when an AI system generates information that appears credible but is factually incorrect or completely fabricated. This happens because AI models rely on statistical predictions rather than true comprehension.
Common Causes of AI Hallucination
- Lack of Real-World Understanding – AI lacks reasoning and relies on training data patterns.
- Data Bias – If AI is trained on biased or incomplete data, it can generate misleading responses.
- Overgeneralization – AI sometimes assumes relationships between unrelated concepts.
- Model Limitations – AI cannot verify real-time facts, leading to outdated or incorrect answers.
The Latest Solutions for Reducing AI Hallucination
1. Reinforcement Learning with Human Feedback (RLHF)
How It Works:
- AI is fine-tuned using human feedback, helping it differentiate between accurate and inaccurate responses.
- AI models learn from human-approved corrections, improving future outputs.
Effectiveness:
- RLHF has significantly improved models like ChatGPT, making them more factually accurate.
2. Improved Data Training and Curation
How It Works:
- AI models are trained on verified, high-quality datasets to reduce misinformation.
- Developers eliminate biased or misleading data before training.
Effectiveness:
- AI models using better-curated datasets exhibit fewer hallucinations.
3. AI Fact-Checking Systems
How It Works:
- AI is integrated with real-time fact-checking databases to validate information.
- Some AI tools, like Google’s Bard, use external sources to verify responses.
Effectiveness:
- Helps minimize errors in AI-generated content.
- However, real-time fact-checking increases processing time.
4. Confidence Scoring Mechanisms
How It Works:
- AI assigns a confidence score to its responses, indicating reliability.
- Low-confidence answers can be flagged for review.
Effectiveness:
- Users can assess AI reliability before accepting responses.
5. Hybrid AI Models (AI + Human Review)
How It Works:
- AI-generated content is reviewed by human experts before publication.
- AI acts as an assistant rather than an independent decision-maker.
Effectiveness:
- Reduces errors in legal, medical, and financial AI applications.
- Slower compared to fully automated AI.
Comparison of AI Hallucination Reduction Techniques
Solution | How It Works | Effectiveness | Challenges |
---|---|---|---|
RLHF | AI learns from human feedback | High | Requires human intervention |
Better Data Training | Uses verified datasets | Moderate | Data bias still possible |
Fact-Checking Systems | Cross-checks real-time information | High | Slows AI responses |
Confidence Scoring | Assigns reliability scores to answers | Moderate | AI may still misinterpret data |
Hybrid AI Models | AI + Human review process | High | Slower than full automation |
The Future of AI Hallucination Reduction
Companies like OpenAI, Google, and Meta are actively improving AI accuracy through advanced training methods. Future developments may include:
- Self-correcting AI models capable of detecting and correcting their hallucinations.
- Stronger real-time fact-checking integrations for AI-generated content.
- Better multimodal AI models that use text, images, and audio to verify information before generating responses.
FAQs
1. Can AI Hallucination Be Completely Eliminated?
No, but continuous improvements in training, fact-checking, and human oversight can greatly reduce its occurrence.
2. Why Do Large Language Models (LLMs) Hallucinate?
LLMs predict responses based on patterns in data. Without true understanding, they may invent information.
3. How Can Businesses Prevent AI Hallucination?
Businesses can:
- Use AI models with fact-checking capabilities.
- Implement human oversight in AI-generated reports.
- Train AI on high-quality, verified data.
4. Are AI Hallucinations Dangerous?
They can be, especially in medicine, law, and finance where accuracy is critical. Misleading AI outputs can cause misinformation.
5. What’s the Best Solution for AI Hallucination?
A combination of RLHF, fact-checking, and human review offers the best accuracy.
AI hallucination remains a challenge, but new solutions are making AI more accurate and reliable. Reinforcement Learning with Human Feedback (RLHF), AI fact-checking systems, confidence scoring, and hybrid AI models are leading the way in minimizing false outputs.
More TechResearch’s Insights and News
AI in Fintech: Enhancing Efficiency & Security in 2025
AI in Next 5 Years: How Will Artificial Intelligence Evolve?