How Synthetic Data Enhances Privacy in AI & Big Data
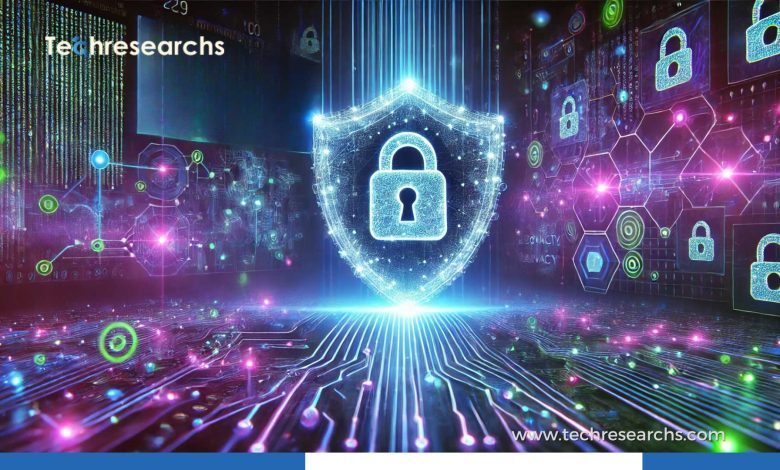
In today’s data-driven world, synthetic data is revolutionizing how businesses and researchers protect privacy in AI and big data applications. As data privacy regulations tighten, synthetic data offers a way to maintain data utility while ensuring compliance. But what exactly is synthetic data, and how does it enhance privacy? Let’s explore its role in securing sensitive information, improving AI model training, and driving innovation.
What is Synthetic Data?
Synthetic data is artificially generated data that mimics real-world datasets but does not contain personally identifiable information (PII). It is created using algorithms, statistical models, and machine learning techniques. Unlike traditional anonymization methods, synthetic data retains the statistical properties of real data, making it highly valuable for AI and big data applications.
Primary Benefits of Synthetic Data for Privacy
- Eliminates Privacy Risks – Since synthetic data contains no real personal data, it reduces the risk of data breaches.
- Regulatory Compliance – Helps organizations comply with privacy laws like GDPR, CCPA, and HIPAA.
- Improves AI Model Performance – Enables AI models to train on diverse, high-quality datasets without legal constraints.
- Enhances Data Sharing – Allows secure data sharing between organizations and industries without exposing sensitive details.
Why is Synthetic Data Important for AI & Big Data Privacy?
1. Protecting Personal Data in AI Training
AI models require vast amounts of data to learn effectively. However, using real-world data can lead to privacy risks, especially in healthcare, finance, and retail. Synthetic data ensures that AI systems can be trained on accurate, privacy-compliant datasets without exposing personal information.
2. Meeting Data Privacy Regulations
Stringent data regulations such as GDPR and CCPA impose strict guidelines on handling personal data. Companies can use synthetic data to avoid legal penalties while still leveraging big data analytics.
3. Preventing Data Breaches
Cyberattacks targeting personal data have increased. Synthetic data reduces risks since it does not contain actual user information, making it useless for hackers.
4. Enabling Secure Data Sharing Across Industries
Many industries struggle to share real-world datasets due to privacy concerns. Synthetic data provides a privacy-preserving solution that allows organizations to collaborate on AI-driven projects without violating confidentiality agreements.
Applications of Synthetic Data in AI & Big Data
Industry | Use Case | Privacy Benefit |
---|---|---|
Healthcare | Medical research & diagnostics | HIPAA compliance, secure patient data |
Finance | Fraud detection & risk assessment | Protects financial records from breaches |
Retail | Customer behavior analysis | Prevents exposure of real customer data |
Autonomous Vehicles | AI training for self-driving cars | Allows safe testing without real-world risks |
Cybersecurity | Threat detection algorithms | Enhances security models without exposing sensitive data |
Challenges of Using Synthetic Data for Privacy
While synthetic data offers numerous advantages, it also comes with some challenges:
- Data Accuracy – Poorly generated synthetic data may lack the nuances of real-world datasets.
- Bias in Data Generation – If training data has biases, synthetic data may replicate them, leading to unfair AI decisions.
- Computational Costs – Generating high-quality synthetic data requires advanced algorithms and computational power.
Best Practices for Implementing Synthetic Data in AI & Big Data
- Ensure High-Fidelity Data Generation – Use advanced AI models to create realistic synthetic datasets.
- Validate with Real Data – Regularly compare synthetic data with real-world samples to maintain accuracy.
- Follow Ethical AI Guidelines – Avoid bias and ensure fairness in data generation.
- Integrate with Privacy-Enhancing Technologies (PETs) – Combine synthetic data with homomorphic encryption and differential privacy for enhanced security.
Future of Synthetic Data in AI & Big Data Privacy
The adoption of synthetic data is expected to rise as organizations seek privacy-friendly AI solutions. Advances in generative AI, such as GANs (Generative Adversarial Networks), will further improve the quality and utility of synthetic datasets. Governments and enterprises alike are investing in synthetic data technologies to address growing privacy concerns.
FAQs
1. How does synthetic data ensure privacy in AI?
Synthetic data does not contain real-world personal information, reducing the risk of data breaches while still maintaining data utility for AI training.
2. Can synthetic data replace real data?
While it can supplement real data, synthetic data should be validated alongside real datasets to maintain accuracy and reliability.
3. Is synthetic data compliant with GDPR and CCPA?
Yes, since synthetic data does not contain personally identifiable information, it helps businesses comply with GDPR, CCPA, and other privacy laws.
4. What industries benefit most from synthetic data?
Industries like healthcare, finance, retail, cybersecurity, and autonomous vehicles leverage synthetic data for AI development and privacy protection.
Synthetic data is transforming the landscape of AI and big data by enhancing privacy, improving compliance, and mitigating security risks. As more industries adopt this technology, the balance between innovation and privacy protection will continue to evolve. Organizations looking to implement AI-driven solutions must consider synthetic data as a critical tool for ensuring ethical and privacy-compliant AI development.
More TechResearch’s Insights and News
Synthetic Biology & Biomedical Engineering:A Strong Alliance