Machine Learning in Cybersecurity: A New Defence Era
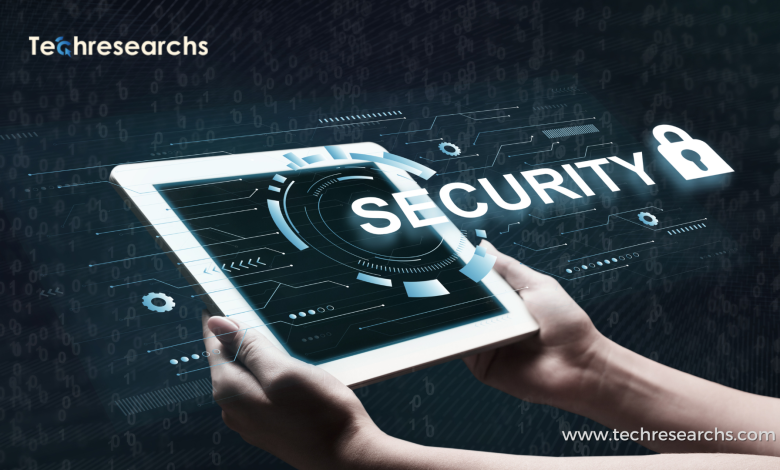
In today’s digital age, cybersecurity has become one of the most critical concerns for businesses and individuals alike. With increasing threats from cybercriminals, traditional security methods are no longer enough. This is where machine learning in cybersecurity is making a significant impact. By leveraging machine learning algorithms, security systems can detect, respond to, and prevent cyberattacks in ways that were previously impossible. In this article, we will explore how machine learning is revolutionizing cybersecurity and why it is the future of digital defence.
What is Machine Learning in Cybersecurity?
Machine learning is a subset of artificial intelligence (AI) that allows systems to learn from data and improve over time without explicit programming. In the context of cybersecurity, machine learning enables systems to recognize patterns, detect anomalies, and predict potential threats based on historical data.
By analysing vast amounts of data, machine learning models can identify subtle differences between normal and malicious behaviour. These models can then adapt and enhance their detection abilities, improving as they are exposed to more data. This makes them invaluable in protecting systems from a wide range of cyber threats, including malware, ransomware, phishing attacks, and network intrusions.
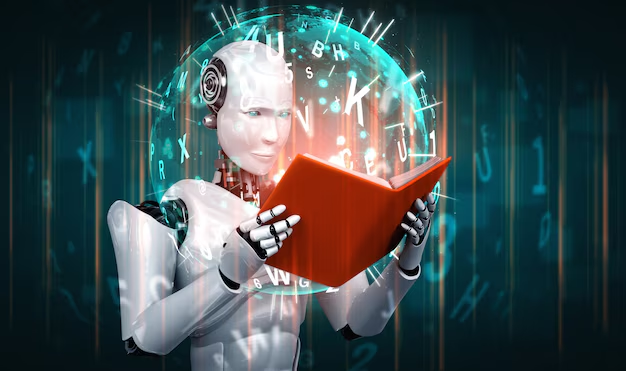
How Machine Learning is Shaping the Future of Cybersecurity
Machine learning is transforming cybersecurity in several ways. Let’s look at some of the most important applications:
- Advanced Threat Detection
Traditional cybersecurity tools often rely on rule-based systems to detect threats. However, these systems can only recognize known patterns. Machine learning, on the other hand, can detect both known and unknown threats by analysing patterns in data. For example, machine learning algorithms can identify unusual traffic patterns in a network and flag them as potential threats. They can also spot new types of malwares that do not match existing signature-based definitions, providing protection against zero-day attacks (attacks that exploit vulnerabilities that are not yet known). - Automated Response and Mitigation
The speed at which cyberattacks occur makes it difficult for security teams to respond manually in real time. Machine learning can help by automating response actions. When a threat is detected, machine learning systems can trigger predefined actions to mitigate the risk. For instance, if a system detects abnormal login behaviour, it can automatically block access, notify administrators, and even roll back any changes made by the malicious actor. This automation significantly reduces the time between threat detection and response, minimizing potential damage. - Phishing Detection
Phishing remains one of the most common methods used by cybercriminals to steal sensitive information. Machine learning has proven highly effective in identifying phishing attempts by analysing email content, links, and user behaviour patterns.AI-powered phishing detection tools can examine incoming emails, determine whether they contain suspicious links or malicious attachments, and flag them as phishing attempts. Over time, these tools learn to recognize new phishing techniques, improving their accuracy and reliability. - Fraud Prevention
Fraud detection systems are crucial for financial institutions, e-commerce platforms, and any organization handling sensitive transactions. Machine learning is used to identify fraudulent activity by analysing transaction patterns. For example, machine learning can detect sudden spikes in transactions, unusual locations, or discrepancies in purchasing behaviour, signalling potential fraud. The system can then flag the transactions for review, or automatically block them, preventing financial losses. - Behavioural Analytics
Machine learning can be used to establish baselines for normal user behaviour, allowing systems to detect anomalies in real-time. By understanding how a user typically interacts with systems, AI can quickly identify unusual behaviour that may indicate an insider threat or account compromise. For example, if a user’s account begins to access sensitive files or conduct suspicious transactions, machine learning algorithms can raise an alert and initiate an investigation.
Benefits of Machine Learning in Cybersecurity
The benefits of machine learning in cybersecurity are profound and far-reaching. Here are some key advantages:
- Improved Accuracy
Traditional security systems may miss subtle signs of an attack. Machine learning models, however, continuously improve and learn from new data, allowing them to detect more complex threats with greater accuracy. As the system encounters new types of attacks, it adapts, becoming more effective over time. - Proactive Threat Prevention
Machine learning allows for the identification of potential threats before they occur. By analyzing historical data and recognizing patterns, machine learning models can predict and prevent future attacks. This predictive capability is a major shift from reactive security measures, where threats are only addressed after they’ve already caused harm. - Cost Savings
By automating threat detection and response, machine learning reduces the need for human intervention, saving time and resources. Automated systems can handle the majority of the workload, allowing security teams to focus on more complex issues. Moreover, early detection and rapid response minimize the damage caused by cyberattacks, leading to fewer financial losses. - Scalability
As businesses grow and their networks become more complex, it becomes increasingly difficult to monitor and secure every aspect of their digital infrastructure manually. Machine learning systems can scale effortlessly, processing massive amounts of data in real-time without compromising on accuracy. This makes them ideal for organizations of all sizes.
Challenges and Considerations
While machine learning offers powerful benefits, it’s not without challenges:
- Data Privacy and Security
Machine learning algorithms require vast amounts of data to learn and improve. However, ensuring the privacy and security of this data is essential. Any breach of the data used to train machine learning models could compromise the system’s integrity and effectiveness. - Adversarial Attacks on AI Systems
While machine learning can detect cyber threats, cybercriminals are also adapting their tactics. Adversarial attacks are attempts to trick AI systems into making incorrect predictions by feeding them misleading data. Ensuring that machine learning models are resistant to these attacks is a critical concern for security experts. - Training and Resources
Implementing machine learning in cybersecurity requires skilled professionals and significant computational resources. Organizations need to invest in training their teams to effectively manage and implement machine learning solutions.
The Future of Machine Learning in Cybersecurity
As machine learning continues to evolve, its role in cybersecurity will only become more prominent. We can expect to see even more advanced algorithms capable of detecting increasingly sophisticated threats, better integration with other cybersecurity tools, and more real-time threat response capabilities.
Furthermore, as the Internet of Things (IoT) expands and more devices become interconnected, machine learning will be essential in protecting these devices from emerging cyber risks. The future of cybersecurity is smart, proactive, and driven by AI-powered machine learning models.
More Related Blogs: Future of the Cybersecurity industry: More Bots, More Cash, and Limited Talent – TechResearchs
Machine learning in cybersecurity marks a new era of defence against digital threats. Its ability to detect, predict, and respond to cyberattacks in real time has revolutionized the industry. As machine learning continues to evolve, it will play an even more integral role in safeguarding businesses, governments, and individuals from the growing landscape of cyber threats. Organizations that adopt machine learning for cybersecurity will be better positioned to navigate and protect against the challenges of the future.