BlackRock Crypto ETF: Will It Make Bitcoin a Mainstream Asset?
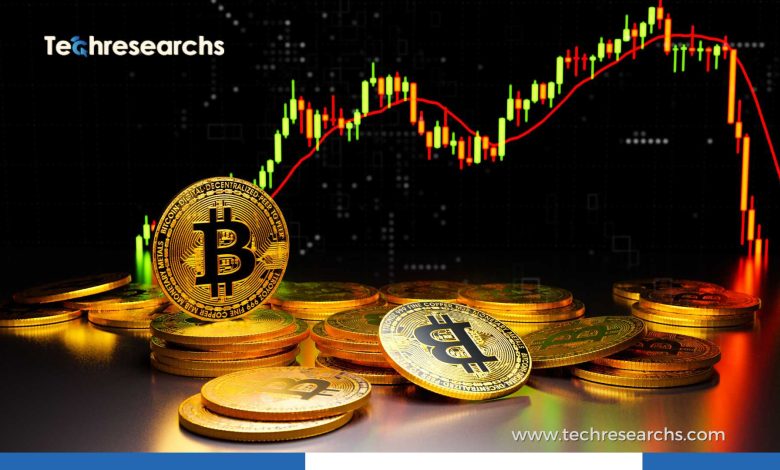
The rise of cryptocurrency exchange-traded funds (ETFs) has been a significant development in the financial industry. With BlackRock, the world’s largest asset manager, showing interest in BlackRock’s Crypto ETF, many investors are wondering if this could be the moment that propels Bitcoin into the mainstream financial market. This article explores BlackRock Crypto ETF involvement in the crypto ETF space and how it may impact the broader adoption of Bitcoin.
What Is a Crypto ETF?
A crypto ETF is an exchange-traded fund that tracks the price of cryptocurrencies, allowing investors to gain exposure to digital assets without directly purchasing them. ETFs are traded on traditional stock exchanges, making them accessible to institutional and retail investors alike.
Crypto ETFs can be categorized into:
- Spot Crypto ETFs – Directly hold cryptocurrency assets and track their market price.
- Futures-based Crypto ETFs – Invest in cryptocurrency futures contracts rather than holding actual digital assets.
- Equity-based Crypto ETFs – Invest in stocks of companies involved in the cryptocurrency ecosystem.
By offering a regulated way to gain exposure to Bitcoin, BlackRock’s Crypto ETF could play a crucial role in driving adoption.
BlackRock’s Role in the Crypto ETF Market
BlackRock has a strong reputation in the financial industry, managing trillions in assets across multiple investment vehicles. Its move toward a Bitcoin ETF suggests increasing institutional confidence in digital assets.
BlackRock’s Bitcoin ETF Filing
In June 2023, BlackRock filed for a spot Bitcoin ETF, aiming to provide investors with direct exposure to Bitcoin’s price movements. This development is seen as a significant step toward mainstream acceptance of cryptocurrency investments. The fund would be managed similarly to traditional ETFs, ensuring investor protection and regulatory compliance.
How BlackRock’s Involvement Differs from Previous Attempts
Several companies have previously sought approval for Bitcoin ETFs, but many-faced regulatory roadblocks. However, BlackRock’s application stands out because:
- It includes a surveillance-sharing agreement with a regulated exchange to address concerns about market manipulation.
- BlackRock’s reputation and experience in ETF management may improve its chances of securing regulatory approval.
- It signals a shift in traditional financial institutions embracing crypto assets.
How Could BlackRock’s Crypto ETF Impact Bitcoin’s Adoption?
1. Increased Institutional Participation
Institutional investors have traditionally been hesitant to invest in Bitcoin due to regulatory uncertainty and market volatility. BlackRock’s involvement may encourage more institutional participation, adding legitimacy to Bitcoin as an asset class. If major institutions begin allocating capital to Bitcoin through ETFs, it could drive demand and price appreciation.
2. Regulatory Approval and Market Confidence
A BlackRock-backed Bitcoin ETF would likely undergo stringent regulatory scrutiny. If approved, it could set a precedent for other asset managers to launch similar crypto-focused investment products. Regulatory clarity often leads to increased investor confidence, which could boost Bitcoin’s adoption across traditional finance.
3. Greater Accessibility for Retail Investors
Currently, investing in Bitcoin requires setting up a digital wallet and navigating cryptocurrency exchanges. A BlackRock ETF would enable investors to gain Bitcoin exposure through traditional brokerage accounts, simplifying the investment process and attracting a broader range of investors.
4. Potential for Market Stabilization
The introduction of institutional-grade investment products could reduce Bitcoin’s volatility by bringing more liquidity and long-term investors into the market. This could help Bitcoin become a more stable and widely accepted store of value.
5. Competition Among ETF Providers
If BlackRock’s Bitcoin ETF gains approval, other financial institutions may follow suit, leading to increased competition. This could drive innovation and result in more cryptocurrency investment products, further integrating Bitcoin into traditional financial markets.
Challenges and Considerations
Despite the potential benefits, there are challenges that come with a BlackRock Bitcoin ETF:
- Regulatory Uncertainty – The SEC has historically been cautious about approving crypto ETFs due to concerns about fraud and market manipulation.
- Market Volatility – While institutional adoption can bring stability, Bitcoin remains a volatile asset.
- Custody and Security – Managing and securing digital assets remains a key concern for institutional investors.
- Investor Education – Many traditional investors may need to learn more about Bitcoin and blockchain technology before adopting crypto-based ETFs.
Market Response and Predictions
Industry experts and analysts have mixed opinions on how the approval of a BlackRock Crypto ETF could impact the market:
- Optimists believe it will drive Bitcoin prices higher as institutional adoption increases.
- Skeptics argue that while an ETF will boost accessibility, it won’t necessarily solve Bitcoin’s underlying volatility issues.
- Regulators remain cautious, ensuring adequate investor protection before approving any crypto ETF applications.
FAQs
1. What is the significance of BlackRock’s Bitcoin ETF?
BlackRock’s involvement in the Bitcoin ETF market signals growing institutional interest, potentially paving the way for mainstream adoption.
2. How does a crypto ETF differ from buying Bitcoin directly?
A crypto ETF allows investors to gain exposure to Bitcoin without directly holding it, reducing security and custody concerns.
3. When will BlackRock’s Bitcoin ETF be approved?
Approval depends on regulatory decisions, with the SEC carefully reviewing crypto ETF applications.
4. Will BlackRock’s Bitcoin ETF impact Bitcoin’s price?
While it may drive institutional investment, Bitcoin’s price is influenced by various factors, including market demand and regulatory developments.
5. Are there risks in investing in a Bitcoin ETF?
Yes, like any investment, Bitcoin ETFs carry risks, including regulatory challenges, market volatility, and potential liquidity concerns.
BlackRock’s entry into the crypto ETF space could be a game-changer for Bitcoin’s mainstream adoption. While regulatory hurdles remain, the potential for increased institutional involvement and accessibility makes this development significant.
For investors interested in Bitcoin but hesitant about managing private keys or navigating exchanges, a BlackRock Bitcoin ETF could be an attractive investment vehicle. However, it is essential to remain aware of the risks, regulatory updates, and overall market conditions before making investment decisions.